Wavelet Transform-Based Mode Decomposition for EEG Signals under General Anesthesia
PEERJ(2024)
摘要
Background Mode decomposition methods are used to extract the characteristic intrinsic mode function (IMF) from various multidimensional time series signals. We analyzed an electroencephalogram (EEG) dataset for sevoflurane anesthesia using two wavelet transform-based mode decomposition methods, comprising the empirical wavelet transform (EWT) and wavelet mode decomposition (WMD) methods, and compared the results with those from the previously reported variational mode decomposition (VMD) method. Methods To acquire the EEG data, we used the software application EEG Analyzer, which enabled the recording of raw EEG signals via the serial interface of a bispectral index (BIS) monitor. We also created EEG mode decomposition software to perform empirical mode decomposition (EMD), VMD, EWT, and WMD operations. Results When decomposed into six IMFs, the EWT enables narrow band separation of the low-frequency bands IMF-1 to IMF-3, in which all central frequencies are less than 10 Hz. However, in the upper IMF of the high-frequency band, which has a center frequency of ≥ 10 Hz, the dispersion within the frequency band covered was widespread among the individual patients. In WMD, a narrow band of clinical interest is specified using a bandpass filter in a Meyer wavelet filter bank within a specific mode-decomposition discipline. When compared with the VMD and EWT methods, the IMF that was decomposed via WMD was accommodated in a narrow band with only a small variance for each patient. Multiple linear regression analyses demonstrated that the frequency characteristics of the IMFs obtained from WMD best tracked the changes in the BIS upon emergence from general anesthesia. Conclusions The WMD can be used to extract subtle frequency characteristics of EEGs that have been affected by general anesthesia, thus potentially providing better parameters for use in assessing the depth of general anesthesia.
更多查看译文
关键词
Electroencephalogram,Variational mode decomposition,Empirical wavelet transform,Wavelet mode decomposition,Intrinsic mode function
AI 理解论文
溯源树
样例
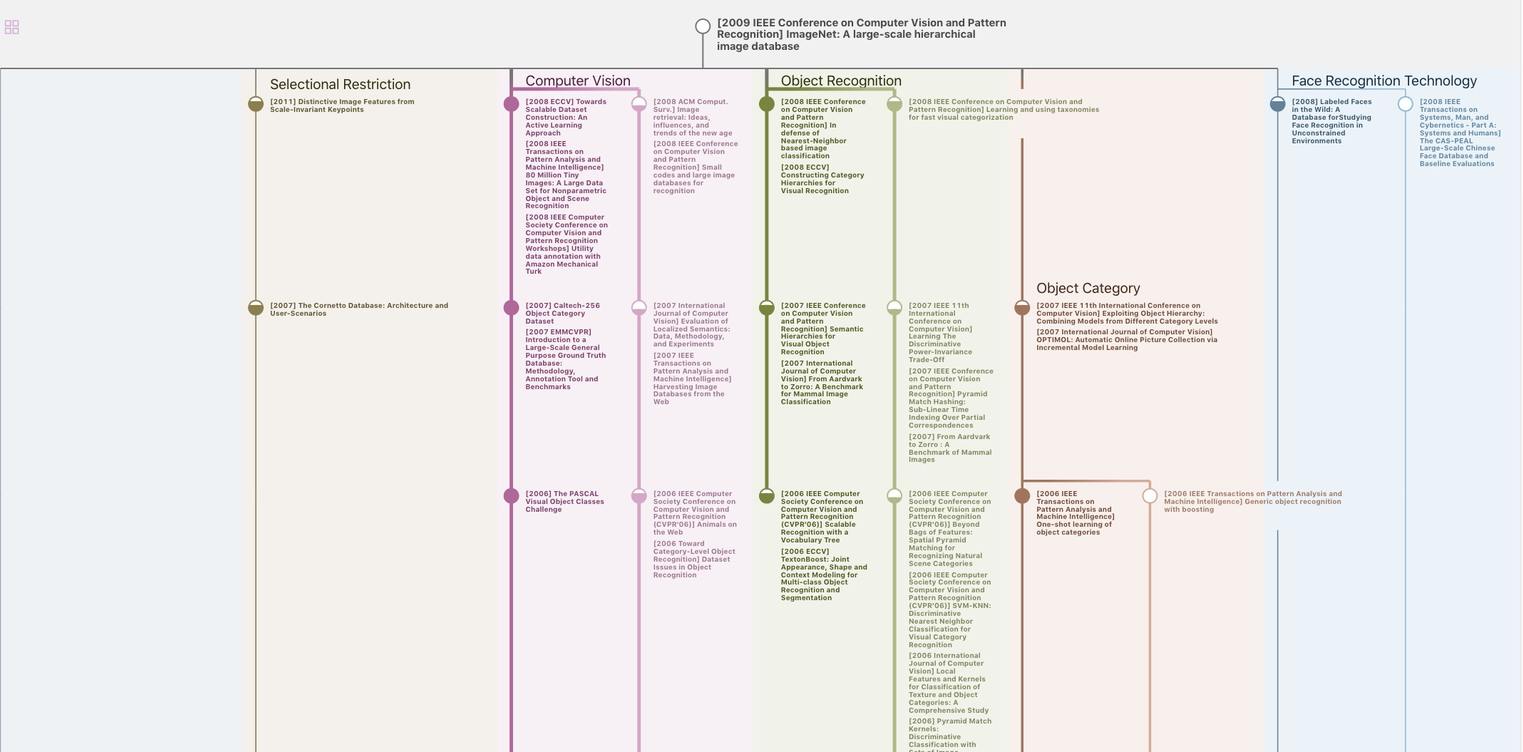
生成溯源树,研究论文发展脉络
数据免责声明
页面数据均来自互联网公开来源、合作出版商和通过AI技术自动分析结果,我们不对页面数据的有效性、准确性、正确性、可靠性、完整性和及时性做出任何承诺和保证。若有疑问,可以通过电子邮件方式联系我们:report@aminer.cn