Double-Exponential Increases in Inference Energy: the Cost of the Race for Accuracy
arxiv(2024)
摘要
Deep learning models in computer vision have achieved significant success but pose increasing concerns about energy consumption and sustainability. Despite these concerns, there is a lack of comprehensive understanding of their energy efficiency during inference. In this study, we conduct a comprehensive analysis of the inference energy consumption of 1,200 ImageNet classification models - the largest evaluation of its kind to date. Our findings reveal a steep diminishing return in accuracy gains relative to the increase in energy usage, highlighting sustainability concerns in the pursuit of marginal improvements. We identify key factors contributing to energy consumption and demonstrate methods to improve energy efficiency. To promote more sustainable AI practices, we introduce an energy efficiency scoring system and develop an interactive web application that allows users to compare models based on accuracy and energy consumption. By providing extensive empirical data and practical tools, we aim to facilitate informed decision-making and encourage collaborative efforts in developing energy-efficient AI technologies.
更多查看译文
AI 理解论文
溯源树
样例
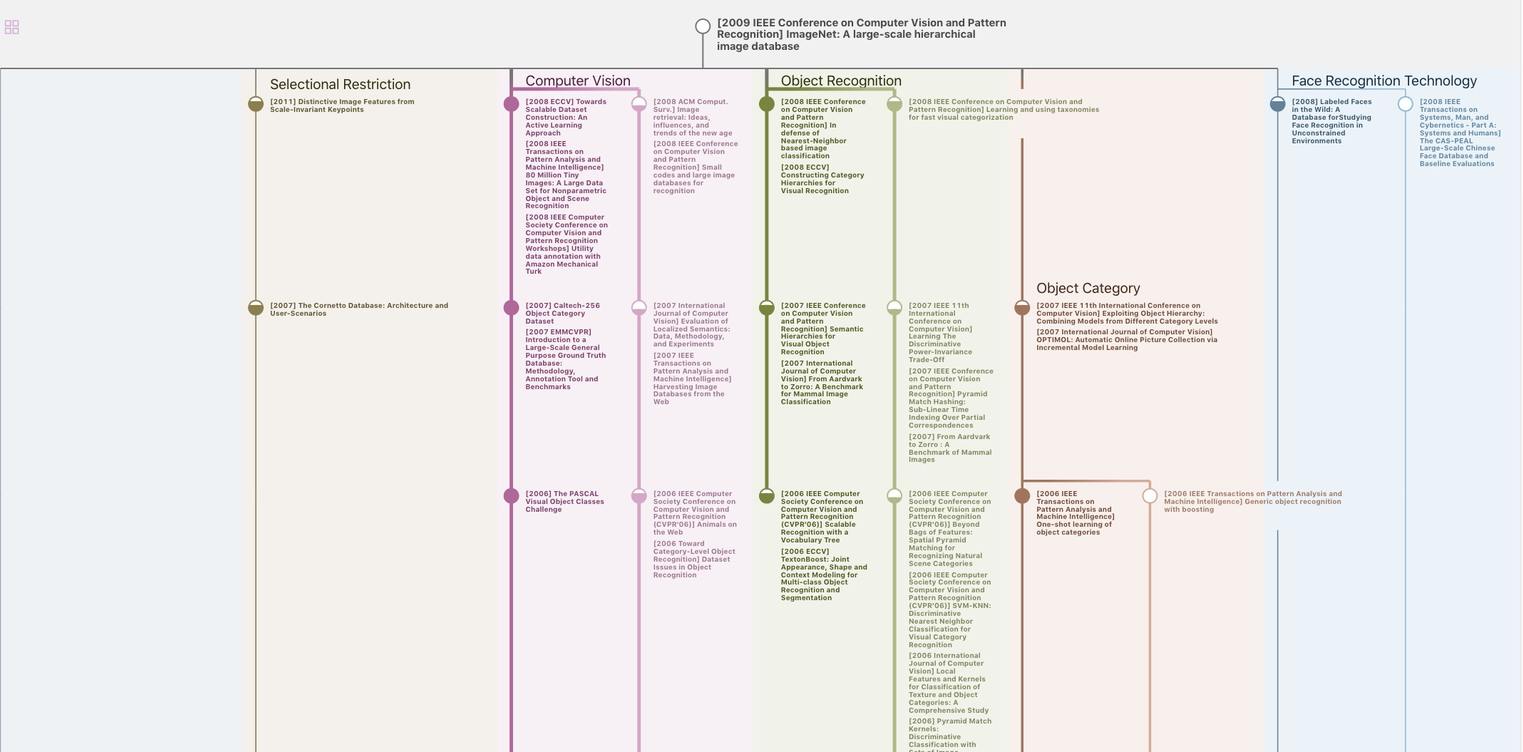
生成溯源树,研究论文发展脉络
数据免责声明
页面数据均来自互联网公开来源、合作出版商和通过AI技术自动分析结果,我们不对页面数据的有效性、准确性、正确性、可靠性、完整性和及时性做出任何承诺和保证。若有疑问,可以通过电子邮件方式联系我们:report@aminer.cn