Spatio-Temporal Dual Graph Neural Networks for Travel Time Estimation
ACM TRANSACTIONS ON SPATIAL ALGORITHMS AND SYSTEMS(2024)
摘要
Travel time estimation is one of the core tasks for the development of intelligent transportation systems. Most previous works model the road segments or intersections separately by learning their spatio-temporal characteristics to estimate travel time. However, due to the continuous alternations of the road segments and intersections in a path, the dynamic features are supposed to be coupled and interactive. Therefore, modeling one of them limits further improvement in accuracy of estimating travel time. To address the above problems, a novel graph-based deep learning framework for travel time estimation is proposed in this paper, namely Spatio-Temporal Dual Graph Neural Networks (STDGNN). Specifically, we first establish the node-wise and edge-wise graphs to respectively characterize the adjacency relations of intersections and that of road segments. In order to extract the joint spatio-temporal correlations of the intersections and road segments, we adopt the spatio-temporal dual graph learning approach that incorporates multiple spatial-temporal dual graph learning modules with multi-scale network architectures for capturing multi-level spatial-temporal information from the dual graph. Finally, we employ the multi-task learning approach to estimate the travel time of a given whole route, each road segment and intersection simultaneously. We conduct extensive experiments to evaluate our proposed model on three real-world trajectory datasets, and the experimental results show that STDGNN significantly outperforms several state-of-art baselines.
更多查看译文
关键词
Travel Time Reliability,Transit Network Design,Spatio-Temporal Data,Travel Behavior,Intelligent Transportation Systems
AI 理解论文
溯源树
样例
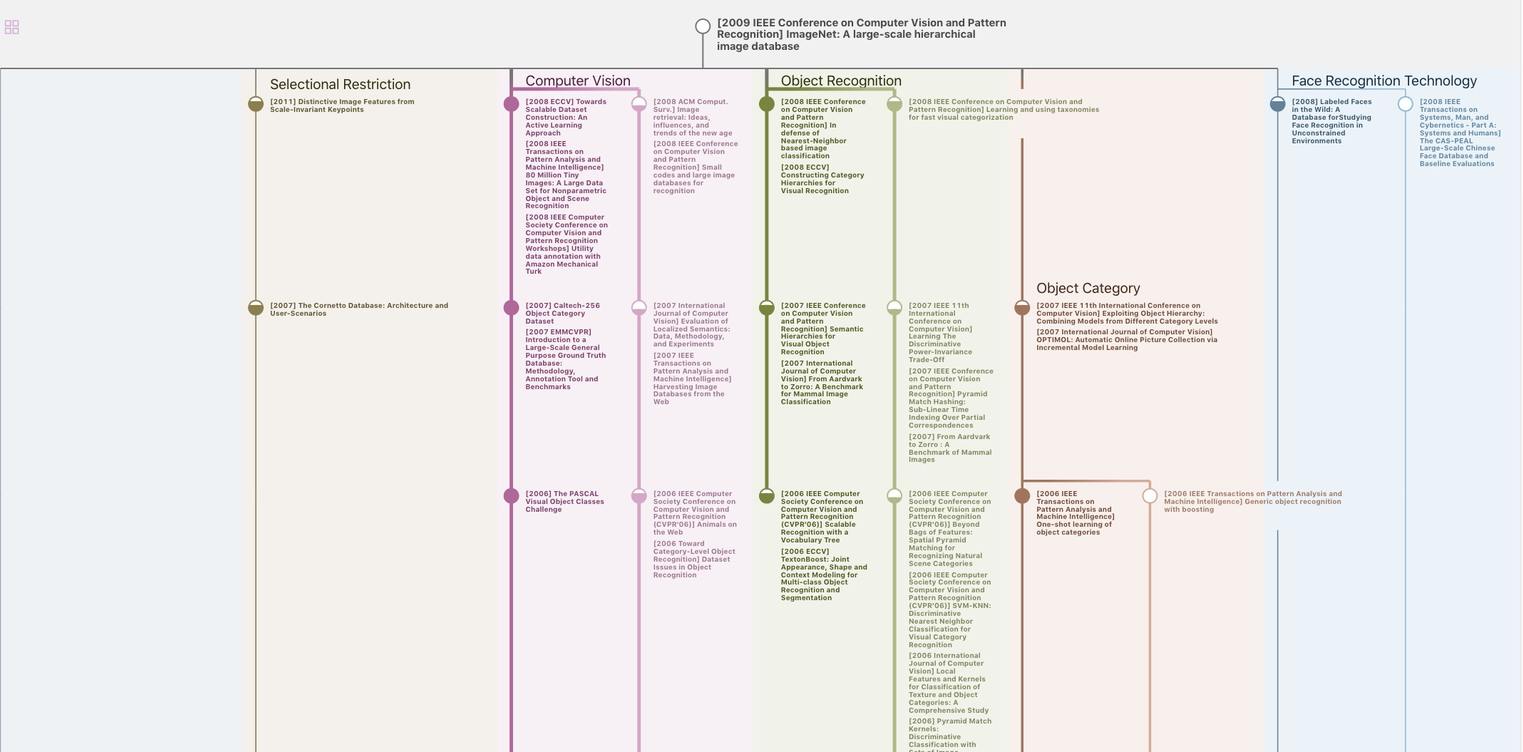
生成溯源树,研究论文发展脉络
数据免责声明
页面数据均来自互联网公开来源、合作出版商和通过AI技术自动分析结果,我们不对页面数据的有效性、准确性、正确性、可靠性、完整性和及时性做出任何承诺和保证。若有疑问,可以通过电子邮件方式联系我们:report@aminer.cn