Evaluating the Effectiveness of Large Language Models in Representing and Understanding Movement Trajectories
CoRR(2024)
摘要
This research focuses on assessing the ability of AI foundation models in representing the trajectories of movements. We utilize one of the large language models (LLMs) (i.e., GPT-J) to encode the string format of trajectories and then evaluate the effectiveness of the LLM-based representation for trajectory data analysis. The experiments demonstrate that while the LLM-based embeddings can preserve certain trajectory distance metrics (i.e., the correlation coefficients exceed 0.74 between the Cosine distance derived from GPT-J embeddings and the Hausdorff and Dynamic Time Warping distances on raw trajectories), challenges remain in restoring numeric values and retrieving spatial neighbors in movement trajectory analytics. In addition, the LLMs can understand the spatiotemporal dependency contained in trajectories and have good accuracy in location prediction tasks. This research highlights the need for improvement in terms of capturing the nuances and complexities of the underlying geospatial data and integrating domain knowledge to support various GeoAI applications using LLMs.
更多查看译文
AI 理解论文
溯源树
样例
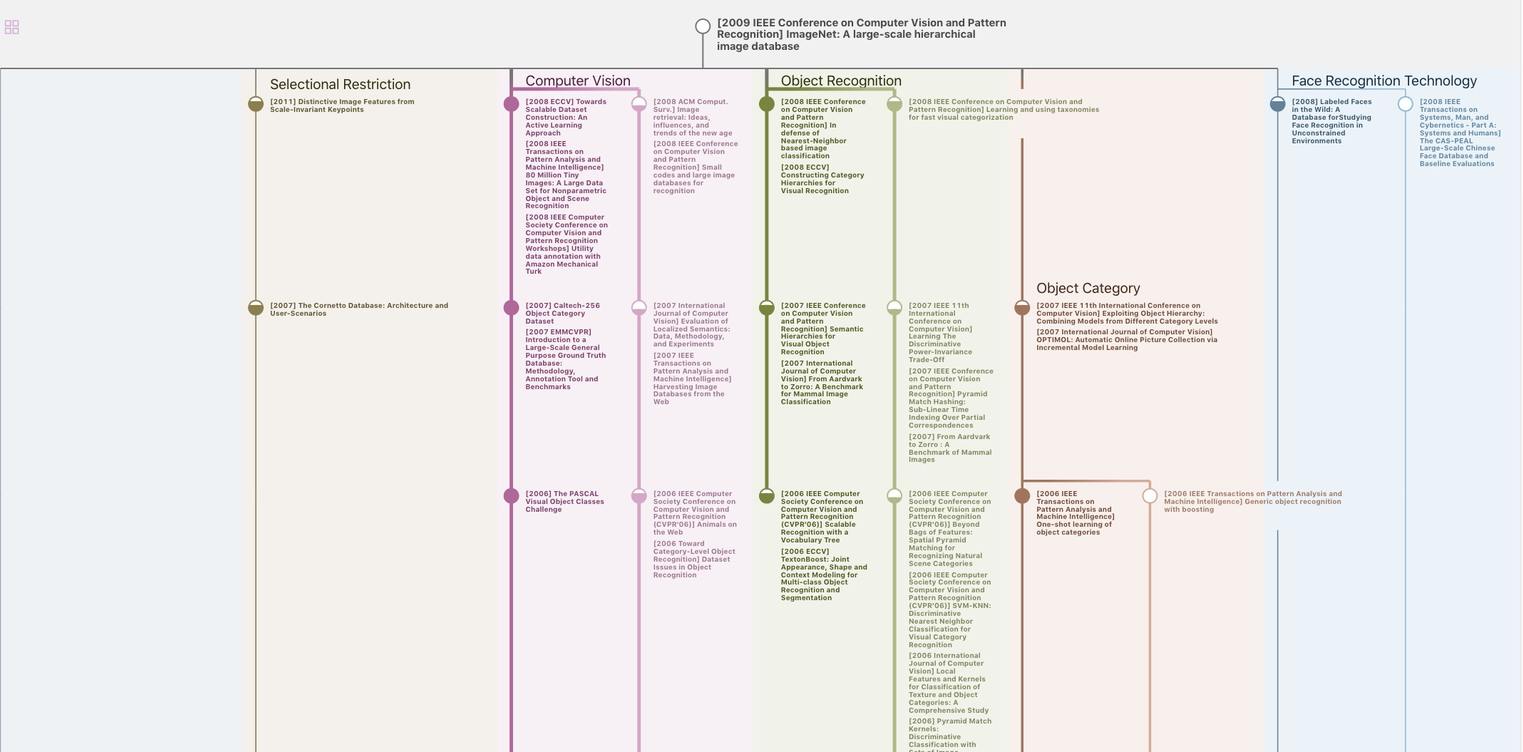
生成溯源树,研究论文发展脉络
数据免责声明
页面数据均来自互联网公开来源、合作出版商和通过AI技术自动分析结果,我们不对页面数据的有效性、准确性、正确性、可靠性、完整性和及时性做出任何承诺和保证。若有疑问,可以通过电子邮件方式联系我们:report@aminer.cn