Digital Twin Enhanced Multi-Agent Reinforcement Learning for Large-Scale Mobile Network Coverage Optimization
ACM Transactions on Knowledge Discovery from Data(2024)
摘要
With the rapid advancement of communication technology and the exponential growth of mobile users, improving network coverage quality and throughput has become increasingly important. In particular, large-scale base station (BS) cooperative optimization has become a highly significant topic. BSs can adjust various parameters for high-quality communication, but automating this optimization remains challenging due to environmental sensitivity and interdependencies. Traditional methods for network optimization are constrained by the intricate nature of real-world environments. Further, reinforcement learning (RL) techniques, which are effective for configuration policies, encounter difficulties in intricate, high-dimensional wireless communication networks, especially in multi-agent cooperative optimization. To overcome these challenges, this paper proposes the Enhanced Multi-Agent Proximal Policy Optimization (EMAPPO), which utilizes the capabilities of the UNet network to extract multi-spatial relationships among a massive number of network elements and employs the DiffPool network to efficiently depict the impact of large-scale action coordination among massive agents on coverage performance. To facilitate evaluation in communication optimization, we further introduce a high-fidelity digital twin-driven mobile network. Extensive experiments validate the effectiveness and superior performance of EMAPPO by utilizing the network digital twin. The results demonstrate significant improvements in signal coverage rate and network throughput compared to the competing methods.
更多查看译文
关键词
Digital twin,Mobile networks,Network optimization,Multi-agent reinforcement learning,Coverage optimization
AI 理解论文
溯源树
样例
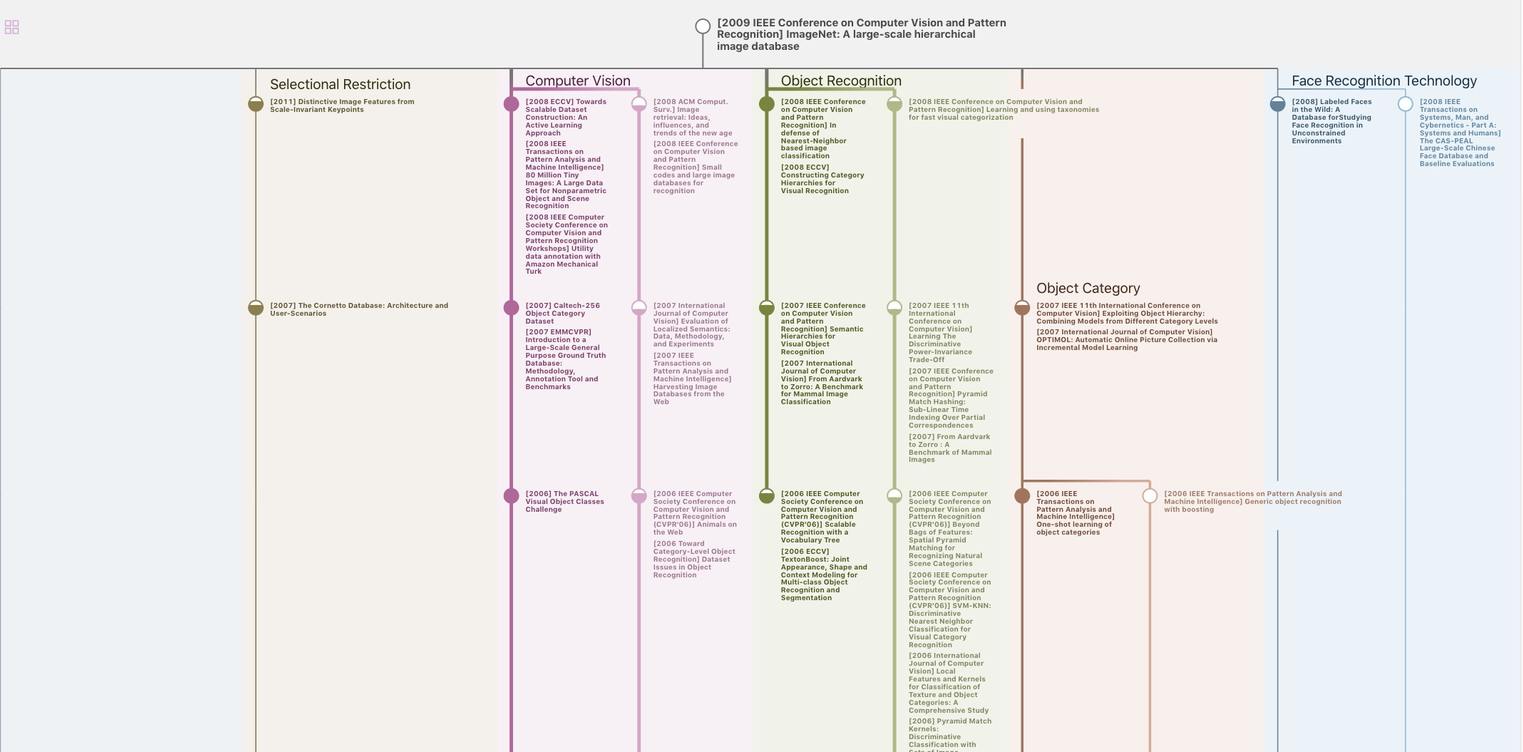
生成溯源树,研究论文发展脉络
数据免责声明
页面数据均来自互联网公开来源、合作出版商和通过AI技术自动分析结果,我们不对页面数据的有效性、准确性、正确性、可靠性、完整性和及时性做出任何承诺和保证。若有疑问,可以通过电子邮件方式联系我们:report@aminer.cn