GeoAI-Enhanced Community Detection on Spatial Networks with Graph Deep Learning
Computers, Environment and Urban Systems(2025)
摘要
Spatial networks are useful for modeling geographic phenomena where spatial interaction plays an important role. To analyze the spatial networks and their internal structures, graph-based methods such as community detection have been widely used. Community detection aims to extract strongly connected components from the network and reveal the hidden relationships between nodes, but they usually do not involve the attribute information. To consider edge-based interactions and node attributes together, this study proposed a family of GeoAI-enhanced unsupervised community detection methods called region2vec based on Graph Attention Networks (GAT) and Graph Convolutional Networks (GCN). The region2vec methods generate node neural embeddings based on attribute similarity, geographic adjacency and spatial interactions, and then extract network communities based on node embeddings using agglomerative clustering. The proposed GeoAI-based methods are compared with multiple baselines and perform the best when one wants to maximize node attribute similarity and spatial interaction intensity simultaneously within the spatial network communities. It is further applied in the shortage area delineation problem in public health and demonstrates its promise in regionalization problems.
更多查看译文
关键词
GeoAI,Community detection,Spatial networks,Graph convolutional networks,Graph attention networks,Neural network embeddings
AI 理解论文
溯源树
样例
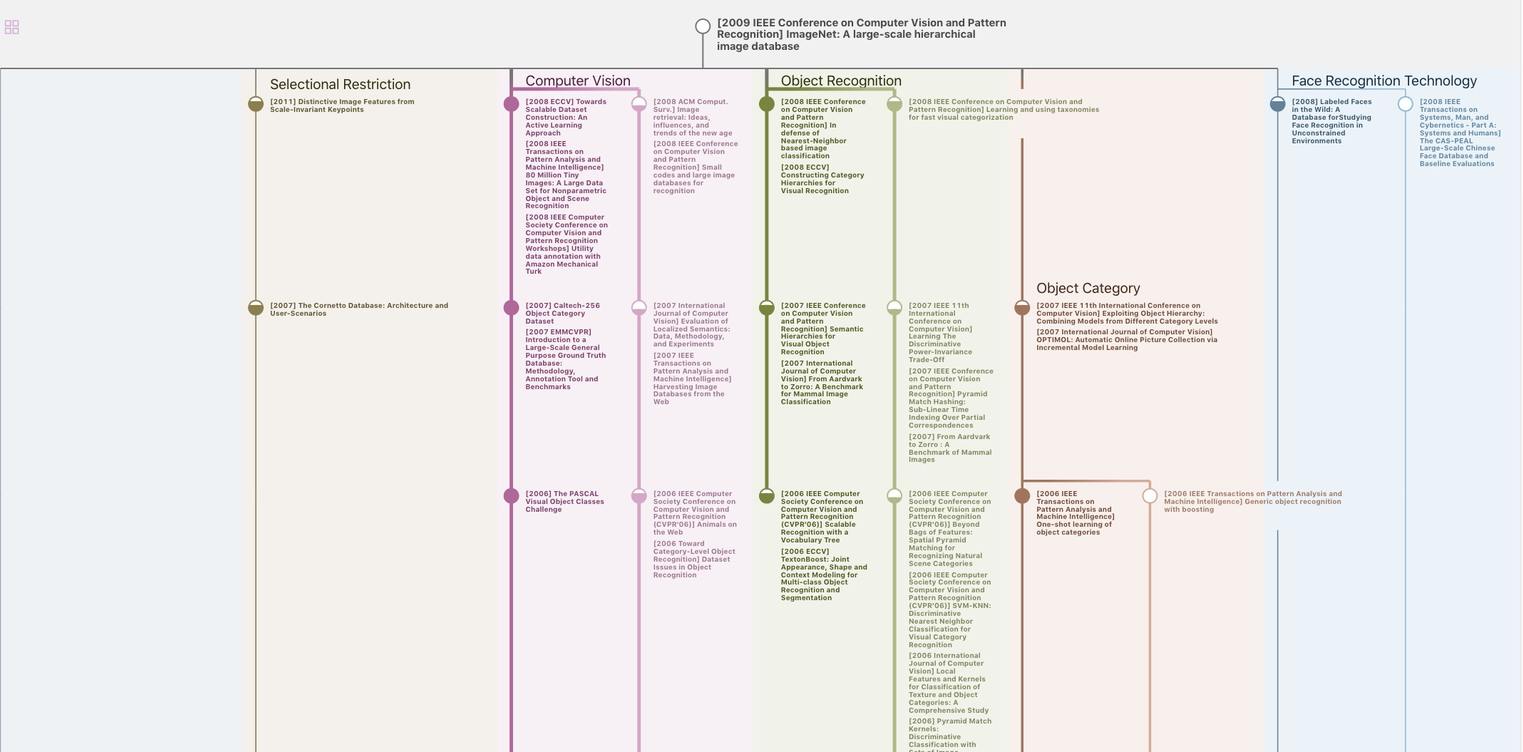
生成溯源树,研究论文发展脉络
数据免责声明
页面数据均来自互联网公开来源、合作出版商和通过AI技术自动分析结果,我们不对页面数据的有效性、准确性、正确性、可靠性、完整性和及时性做出任何承诺和保证。若有疑问,可以通过电子邮件方式联系我们:report@aminer.cn