Gaussian Random Field Approximation Via Stein's Method with Applications to Wide Random Neural Networks
APPLIED AND COMPUTATIONAL HARMONIC ANALYSIS(2024)
摘要
We derive upper bounds on the Wasserstein distance (W1), with respect to sup-norm, between any continuous Rd valued random field indexed by the n-sphere and the Gaussian, based on Stein's method. We develop a novel Gaussian smoothing technique that allows us to transfer a bound in a smoother metric to the W1 distance. The smoothing is based on covariance functions constructed using powers of Laplacian operators, designed so that the associated Gaussian process has a tractable Cameron-Martin or Reproducing Kernel Hilbert Space. This feature enables us to move beyond one dimensional interval-based index sets that were previously considered in the literature. Specializing our general result, we obtain the first bounds on the Gaussian random field approximation of wide random neural networks of any depth and Lipschitz activation functions at the random field level. Our bounds are explicitly expressed in terms of the widths of the network and moments of the random weights. We also obtain tighter bounds when the activation function has three bounded derivatives.
更多查看译文
关键词
Distributional approximation,Gaussian random field,Stein's method,Laplacian-based smoothing,Deep neural networks
AI 理解论文
溯源树
样例
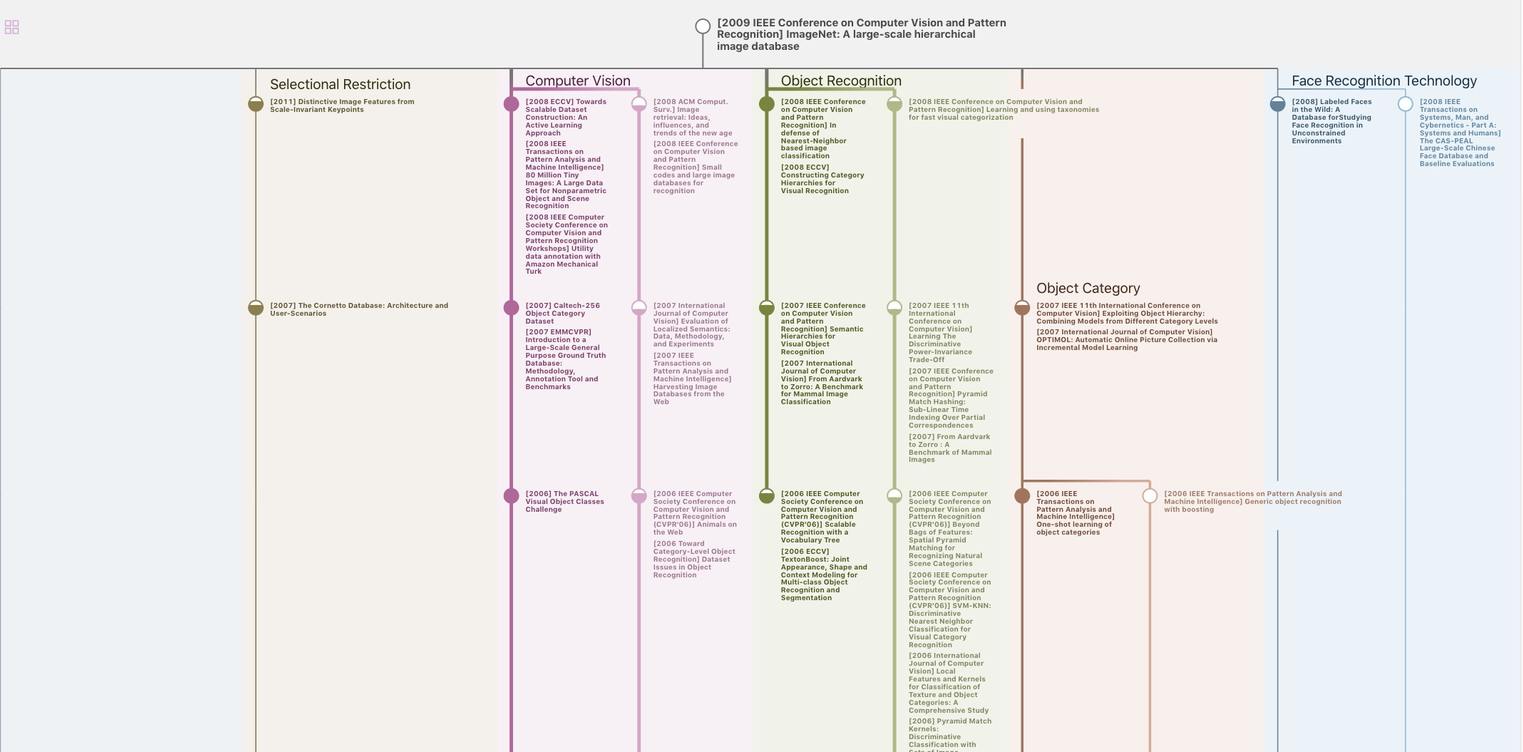
生成溯源树,研究论文发展脉络
数据免责声明
页面数据均来自互联网公开来源、合作出版商和通过AI技术自动分析结果,我们不对页面数据的有效性、准确性、正确性、可靠性、完整性和及时性做出任何承诺和保证。若有疑问,可以通过电子邮件方式联系我们:report@aminer.cn